Monitoring Customer Data With Machine Learning
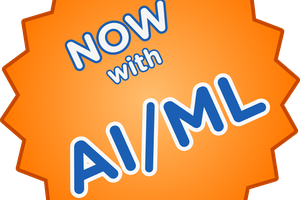
With years of data from thousands of customers available, infrastructure vendors are turning to machine learning to help customers, and themselves, to better manage their systems.
For some time now, infrastructure vendors have been monitoring customer equipment remotely. In days of yore it was with back-to-base telephone links directly connected to the storage area network (SAN) arrays, but these days it's much more likely to be an encrypted stream of data sent over the Internet. When you're spending millions of dollars on systems to keep your most valuable corporate data alive, you want to make sure someone is keeping a close eye on your investment.
And for the vast majority of customers, this has been an unalloyed good thing. Getting remote support from well-trained specialists who can see exactly what's happening on your malfunctioning system gets things fixed quickly when they break, and many situations can be avoided with preemptive maintenance. It's also helpful to the bottom line to encourage customers to buy more disk as the existing arrays start to get full.
With mountains of data being collected, it only makes sense to add some newer analysis techniques that have, somewhat unfortunately, been overhyped as AI.
Those familiar with Nimble Storage (acquired by HPE in 2017) will remember its cloud-based InfoSight monitoring and analytics system that was said by many to be the best thing about Nimble. NetApp has recently added machine learning to its decade-plus collection of AutoSupport data with its cloud-based Active IQ tool. Pure Storage has its Pure1 META system, and Dell EMC has CloudIQ. If you're in the storage game, it seems you have to have a cloud-based monitoring and analytics system of some kind.
This makes a lot of sense for the vendors, and it also makes sense for customers in a way that doesn't for other kinds of tech. With storage systems, the interests of customers and vendors are more-or-less aligned.
Collecting data on the install-base helps the vendors to notice when there are systemic issues with their products. A bug in the operating system can take out a full array of data, so NetApp's Active IQ method of advising admins how many previous installations were rated successful by their owners helps avoid catastrophe. It also builds confidence in the product when you can point to a large database of successful upgrades and performance enhancements.
There's also the potential for industry benchmarking. How well tuned are your systems compared to your peers? This kind of systemic knowledge benefits the entire industry as individual companies strive to be above average. Knowing what good looks like is every bit as useful as avoiding known-bad situations.
Customers benefit both in the short term, with the useful insights from the monitoring, and longer term through improved products. Vendors get near real-time feedback on quality issues they can use to improve their processes.
It's all part of the increasing industrialization of IT. I've long said that it seems to be following a similar path to the auto-industry's discovery of the Toyota Production System that lead to increased quality and decreased costs simultaneously in what was a major shock to US automakers at the time. Making systemic improvements means monitoring and understanding components as part of a systemic whole. In modern IT environments, the system spans organisational boundaries, so gaining insight to what's happening outside your own datacenter can be invaluable.
I find it remarkable that, compared to the near daily scandals over Facebook's handling of data privacy and the all-too-frequent data breaches hitting the news, the infrastructure vendors have done a fabulous job of keeping their customers' data safe and using it for their customers' benefit.
In other parts of the infrastructure stack, we see a reluctance to share data that isn't present at the storage layer. Microsoft's efforts to add more monitoring into Windows 10 has been met with suspicion, and monitoring on mobile devices is similarly decried.
The key seems to be ensuring that customer information is kept within the vendor and only used for purposes that are clearly beneficial to the customers providing their data. It's not normal for storage vendors to share customer monitoring data with third parties like advertisers, data brokers, or governments, and this is backed up with strong contractual obligations to safeguard the data. The same cannot be said for mobile app vendors, or certain handset manufacturers for that matter.
Trust takes time to build, and is all too easily lost. Other parts of the industry could do worse than learning from the storage folks.
This article first appeared in Forbes.com here.